Medical experts can visually identify nodules on a CT scan, but they need to rely on computerized analysis to estimate shape and volume of nodules, as well as their progression over time. It is visually easier to identify nodules than lung tumors, since the former are supposed to have an elliptical shape, while the chromatic aspect of the latter is difficult to distinguish from healthy tissues on a CT image. This difference reflects also on the annotation phase: it will be easier for a non-expert annotator to identify a tumor than a nodule.
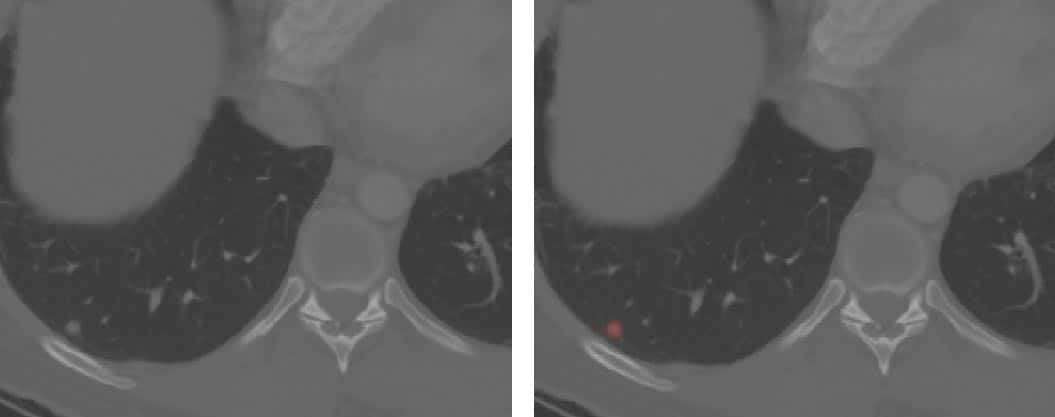
Automatic lung nodules segmentation
Our client needed an automatic process of lung nodules and tumors segmentation to deal with this difficulty in a way that is quick to perform and memory efficient. Our methodology (once built around graph cuts algorithms) currently focuses on Deep Learning with neural networks. Their advantages are multiple: besides offering a much faster segmentation, this is a fully automated solution, able to automatically detect all tumors and nodules, with no need for external intervention. This solution proves to provide a simple and effective answer to many problems of medical segmentation. It is robust and practical, as it solves the challenges fast and efficiently. We at RSIP Vision (and not only us) consider it the best approach to perform nodule and tumor detection and segmentation in lung CT images.
Compared with hand-made nodule segmentation in the lung (performed by an expert), this automatic lung nodules and tumors segmentation method grants results which are very close to the (much slower) manual segmentation. It also enables our client to overcome the difficulty of tumors and healthy tissues appearing on the CT scan in a very similar range of intensity values. You can read here about other projects led by RSIP Vision in the field of pulmonology.