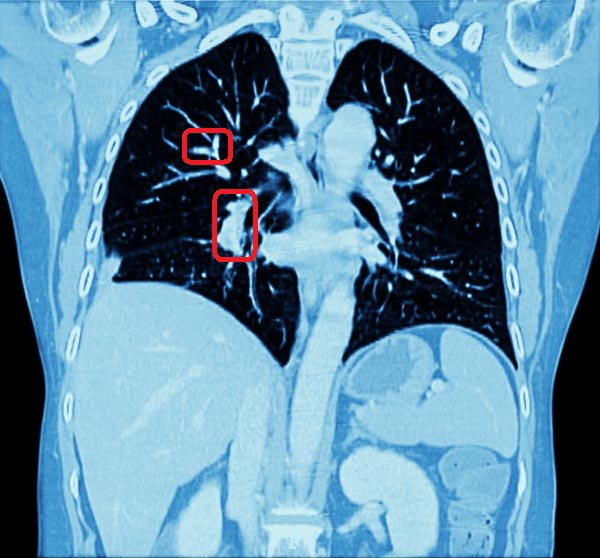
Automated Chest CT Scan Analysis
Computer-aided automated analysis of chest scans can greatly alleviate the downsides of manual analysis, by providing fast, accurate and reproducible detection of lung abnormalities.
With the advances in neural networks and deep learning methodologies, it is now relatively easy to detect dormant lung abnormalities and follow them over successive acquisitions up to disease manifestation. However, prior to the use of an automated image classifier, such as convolutional neural networks, image preprocessing must take place to ensure features extraction is performed in designated position on the lungs.
Analysis towards automated pulmonary disease detection is based on two major steps. At the first stage, the left and right lung are to be separated in the image from the surrounding thoracic tissue. This stage by itself can pose several technical challenges due to variation in lung shapes, patient orientation and cropped X-ray images, while a failure to correctly delineate lung boundaries can result in false information being processed. Several practices exist for the extraction of lung shapes: some of the most common use lung image masks, active contours, and machine-learning based methods. Care must be taken in the boundary detection as abnormalities oftentimes appear in graytone levels similar to those of lung boundaries, with the result of possibly skewing the latter.
Once lung boundaries are extracted, a second stage examines the characteristics of the lung region within the image. Classification of abnormalities based on morphologies, position and gray levels, is routinely done by radiographers. This knowledge can be translated into an autonomous machine decision procedure by training a convolutional neural network to discern between pathologies (a classifier), based on local image characteristics.
Convolutional neural network consists of image convolutional layers to process an image (or regions of), where information between different layers is passed and processed according to a predefined architecture, most often identified empirically. The identification of network architecture for optimal classification is one of the challenges faced by machine learning experts and is subject to the experience of the engineers and the complexity of the problem at hand.
RSIP Vision is a global leading expert in tailor-made machine learning and computer vision algorithms. Our clients around the globe enjoy our accumulated knowledge and experience, gathered from several decades of experience. To get consulting for your project contact us today. To learn more about RSIP Vision’s algorithmic solutions, visit our project page now.