Using aerial images taken by drone, plane or satellite, RSIP Vision can create forestry image processing and analysis software to efficiently determine:
-
Trees detection
Automatic tree detection is the initial phase in many applications. The system is marking the tree center and assign to it the geographic coordinates. One of the major applications here is stock evaluation. The stock figures here are most accurate due to the high detection performance.
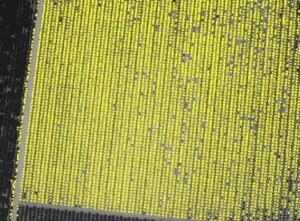
-
Trees classification by type
Tree classification is an automatic post tree detection process. RSIP Vision is using to most advanced techniques like Deep Learning to ensure accurate tree classification even when the image has suffered from corruption and variations of intensity and tone (white balance). It can work with both RGB and CIR images.
-
Tree health
Variations in CIR and NDVI index shows growing status. Since RSIP Vision is supporting both CIR and RGB, this information is fine registered and calculated to point accurately the problematic elements (tree or crop).
-
Stem density
The amount of trees per area unit. This figure can be derived from both tree detection and row detection procedures.
-
Tree crown (canopy) size
One of the major application based on tree detection is crown (canopy) size detection. Some researches show a correlation between the stem size and its canopy. The larger the canopy, the stem is bigger – it yields more wood.
-
Distribution of tree crown diameters
A statistic parameter that shows the general quality of the forest section. It is an application of the crown (canopy) measurement capability. Generally, a distribution with some peak toward larger sizes (larger canopies) shows that more wood can be obtain from the forest section.
-
Young forest planting quality detection
Young trees planting status is most critical. Only the first 3 month is the time gap that re-planting is possible (when the originally planted trees did not grow). There are 2 challenging procedures associated here:
– Young planted trees failure: the detection process impose many difficulties and challenges. The young trees are usually very small and have a small footprint in the aerial image. In addition, weeds and other specious is surrounding the young trees. RSIP Vision’s powerful algorithms for detection and classification are used here.
– Re-planting process optimization: when young trees failures are detected we challenged with the minimal handling (re-planting) effort. The RSIP Vision’s optimization is based on grouping nearby replanting area segments and creating some minimal travel in between.
-
Yield estimation from trees
At any time, RSIP Vision fast and accurate algorithms and methods for tree detection can derive the yield from an aerial image.
-
Sampling planning (Ground proof verification)
Forest stock evaluation is done, traditionally, using ground team. This team is “sampling” an area – measuring the amount of trees inside. Later, extrapolation is performed to evaluate the full forest wood stock. This method is not accurate and is highly depended on human skills and the selected samples areas. RSIP Vision, can assist with this process by suggest areas which reflect the average tree density. It may use to verify the automatic procedures.
-
Supporting RGB and CIR images for tree detection
RSIP Vision’s algorithms can work with any set or subset of gray and color channels.
This automatic tree detection and recognition enables more accurate decision making, saving thus time and costs, particularly in hard-to-reach areas.
Consult with us now for any forestry and precise agriculture image processing project.