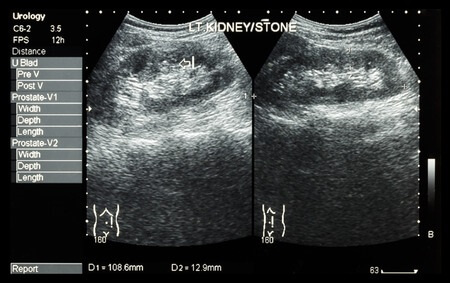
The use of ultrasound in these kidney stone destruction enables exploiting visual information for automatic segmentation and tracking of kidney stones, finally leading to their ablation. Vision-based algorithms can be used to mechanically control the beam’s activation by sending feedback on the position and shape of stones. Kidney stones are rather stationary in the time scale of a treatment, therefore segmentation and tracking are done to compensate respiratory or involuntary motion of patient, ensuring that only intended regions are affected.
There are few strategies for accurately segment kidney stones in ultrasound images. One popular choice would be to study the shape and appearance of stones in ultrasound images by machine learning techniques. These techniques requires that supervised information (manual segmentation) be performed in the learning stages. A definition of the kidney features are made by the algorithm developer and subsequently extracted from each segmented stone image. This allows the computer ‘to learn’ stone kidney appearance as regards the features defined. In terms of algorithmic definition, learning is just adjustment of weights in a statistical model such that the human result and those of the machine are minimized. In the case of kidney stones, we want to minimize the difference between the contour marked by experts and that made automatically by the machine.
One other possibility for segmentation and tracking of kidney stones would be to employ template matching. Similarly to the machine learning example, template matching requires knowledge, or an example, or the appearance of a kidney stone. In contrast to machine learning, template matching can work using an example of a stone derived from the current patient’s ultrasound image. However, this requires that the operator manually segments a kidney stone at the beginning of the operation, or rely on the statistics of a previous example. Other methods include the generation of probabilistic maps of stone positions, which form as candidate search regions for the use of accurately boundary or surface matching.
Using the segmented stones, patient motion compensation can be employed. By motion compensation we mean here that the visual ultrasound information is used to stabilize the high intensity ultrasound machine to operate at a specified point.
With the advancement in AI-based and vision-based algorithms, we can expect automatic procedures like kidney stone destruction be done more frequently. The use of robotic arms controlled by visual information is becoming a common practice in surgery and is expected to be part of ordinary routines in clinics. At RSIP Vision, we specialize in crafting tailor-made vision-based and machine learning algorithms for clinical applications. Our algorithms have been employed in the industry for over 25 years. Consult us and we will help you turn your project into a success.